The Real Challenge in Social Network Data Science
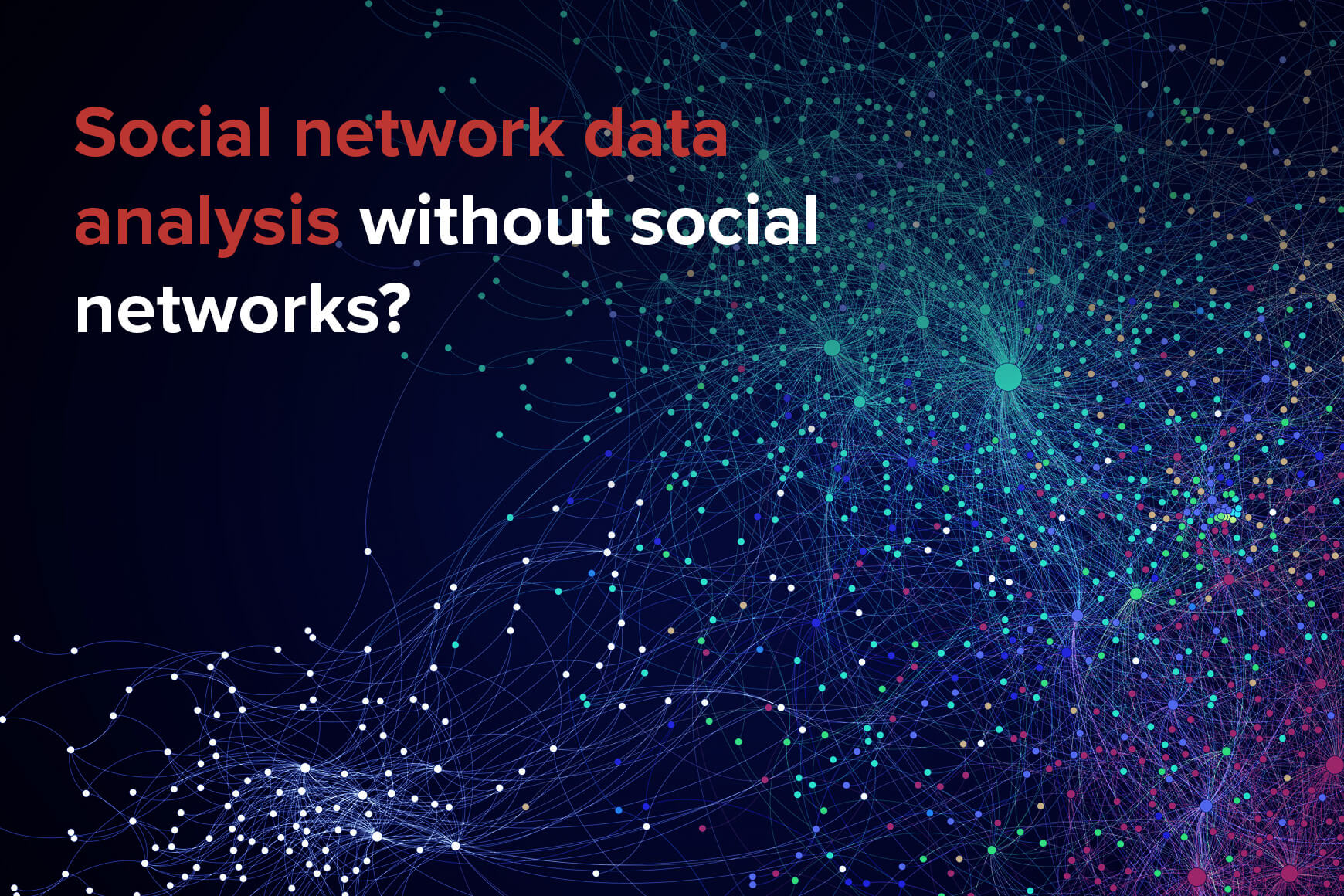
As even the slowest thinkers of our current era have already recognized, social networks and related phenomena, defined in the broadest possible terms, are a great source of insight into human behaviour and preferences as well as a vital resource for data analysis and predictive modelling.
The key phenomenon in predictive modelling based on social network data is called assortativity: the natural tendency of a network’s nodes to develop connections to other nodes sharing similar attributes. We are all familiar with it from our own social networks: it consists of people from the same family, town or generation and people with similar education, professions or hobbies or in similar life situations. We meet such people disproportionately more often than the rest of humanity. It is natural.
The straightforward way to predict the attributes or behaviour of an individual based on his social network is to aggregate the prevalent patterns of his network neighbourhood. There are plenty of algorithms to extract this knowledge. Some are classics, such as graph search and nearest neighbours; others have come along rather recently, including semi-supervised and deep learning approaches. This, however, is not the real challenge of the day. The real challenge is to do social network data analysis, where there is no social network at all. Surprised? Think about it for a moment. In the real world, it is extremely rare to get real social network data, at least if you do not work for Facebook or the NSA.
Although network data in their explicit form are hardly achievable in practice, the world is full of various social networks, and their structures are implicitly recorded in plenty of data sets. Take common office systems data: the structure of email communication, the lists of meetings and their participants, the logs of various collaborative systems such as git, issue trackers, shared document storage, etc. are endless sources of evidence about people sharing their time, ideas and efforts with each other.
The real challenge in social network data science is to pick the right business domain, to understand the sheer complexity of its data, to recognize how social structures are reflected in the seemingly chaotic log data and, finally, to come up with a set of models and heuristics to recover the social network from the data. When this is done, the rest is simple: just observe how people like you are doing, and you know what is likely to happen to you or to your customers, if you are after things like customer segmentation, for example.