Customer Segmentation in Banking
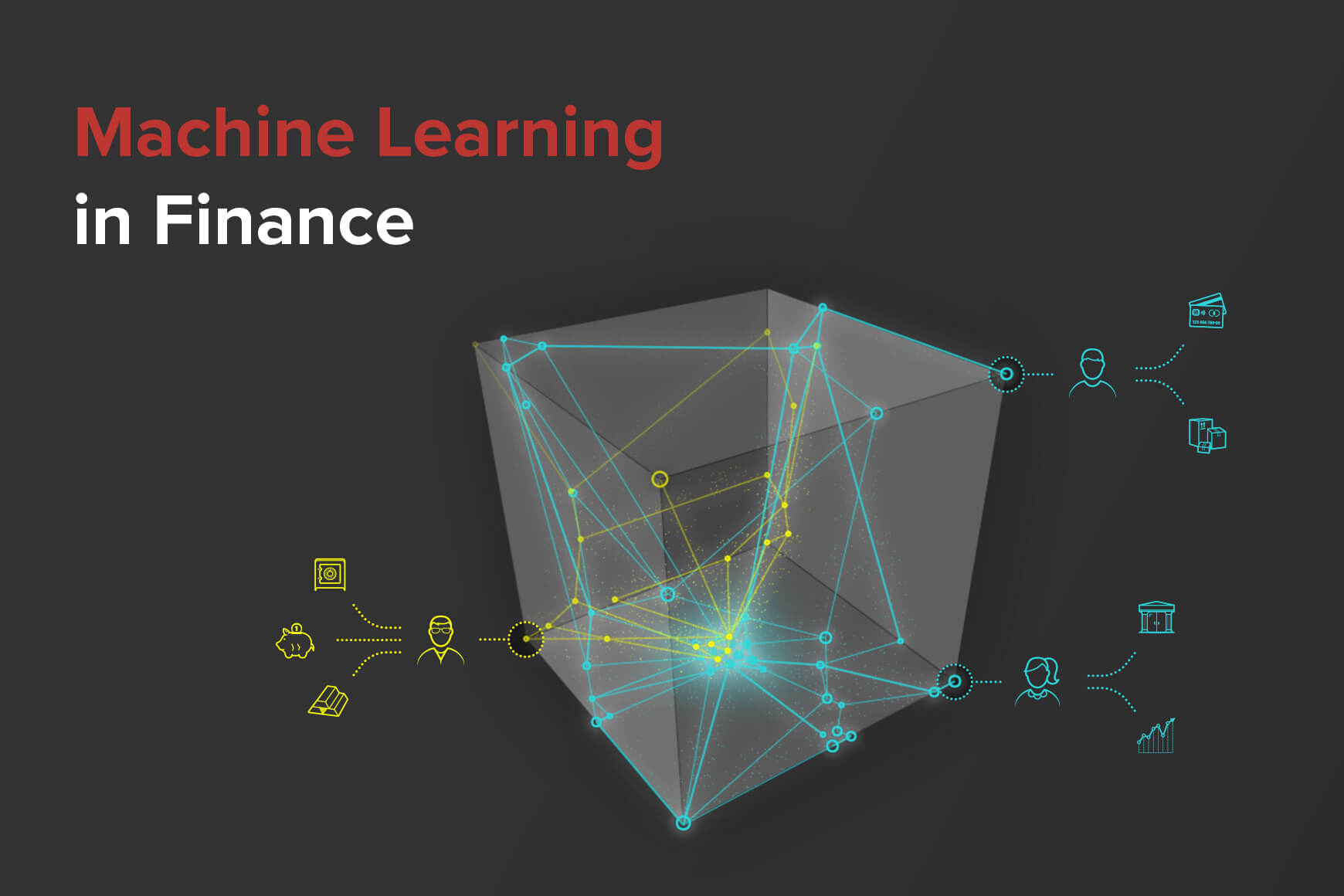
Every company doing business in retail has been dealing with customer segmentation, and so have banking organizations trying to describe their client base effectively. Customer segmentation in banking is key to the success of marketing campaigns, product cross-selling and risk scoring. That’s why it’s critical for financial institutions to set up an efficient customer segmentation strategy.
Although finding the right way to customer segmentation in banking is always a very hard task, it is natural because it is necessary to make a general statement about local information. In this article, we’ll sum up the evolutionary steps from the simple intuitive view to data-driven customer clustering and then advanced micro-segmentation using big data in banking.
The Basic Approach to Customer Segmentation in Banking
This is what you can do with elementary SQL queries and every basic BI tool. You aggregate your data in a cube and cut it in several straightforward dimensions to view your client base from the perspectives of:
- Demographic segmentation
- Geographic segmentation
- Product segmentation
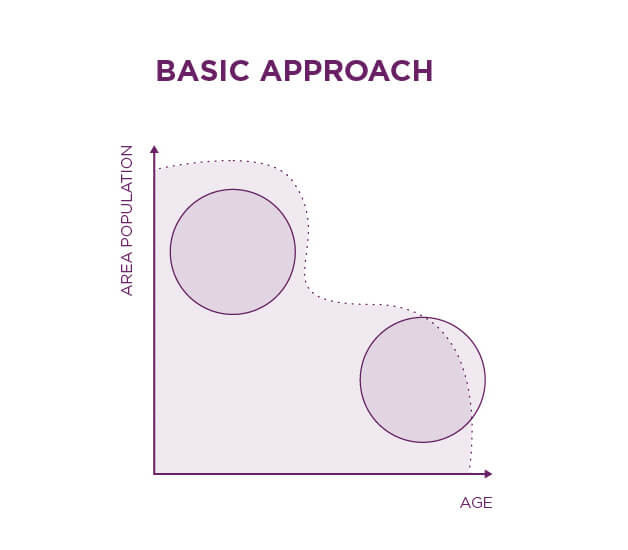
Using this approach, you can tell that men in their thirties who live in urban areas are more likely to have a mortgage than seniors in rural areas. You don’t need any math because it is nearly business intuition. The problem with this approach is that the data don’t show you anything that you don’t already know.
Applying Data Analytics in Customer Segmentation in Banking
If you want to get better insights, you will discover that integrating and using all your customer data is a must. Applying analytics to complex data helps you uncover hidden patterns that are not obvious, like which customer segments tend to get loans and who is a suitable candidate for investment products. You need to start viewing the client segmentation process as being data-driven.
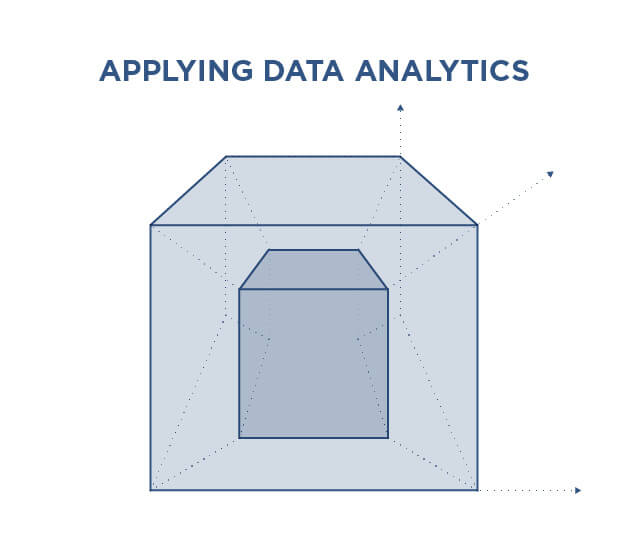
At the same time, with increased data complexity, your customer segmentation gets complicated as well. Now you have to access a lot of data sources and build a data-processing pipeline. New skills and tools such as Python or R are also needed for designing data-driven models. However, the biggest challenge comes at the moment you want to interpret your data-generated results.
New questions arise such as:
- How can I turn the outcomes of my clustering algorithm into usable business segments?
- How can I match data clusters to business labels like savers, investors and loan-takers?
- How many customer segments can I match in a statistically or commercially valid manner?
- Is it enough to create an effective behavioural customer segmentation in banking?
The answers to all these questions lie in your ability to measure the effectiveness of customer segmentation analysis from a strictly business-success point of view. Does your solution bring more value than it costs?
Using Machine Learning in Finance
Once you have successfully applied data-driven customer segmentation, the doors are open to even more complex techniques. Now you can start expanding your teams with data scientists and machine-learning engineers. Their task is to discover new ways to get even deeper insights into your client base.
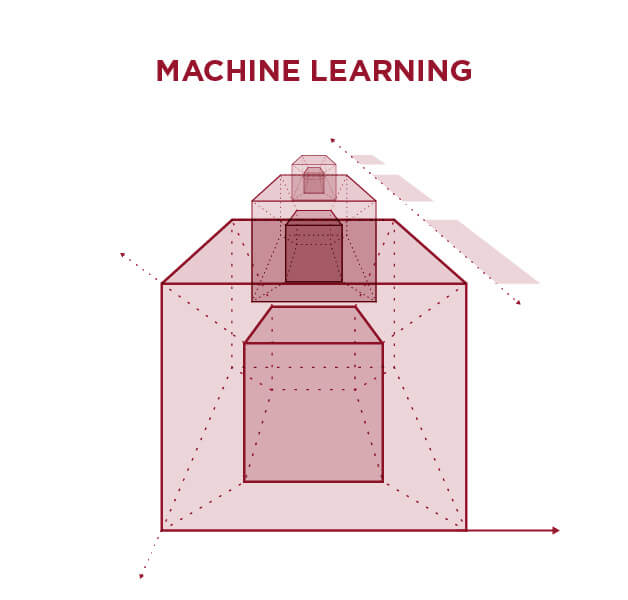
This approach brings you real predictive power. You can use it to analyse the behavioural profiles of your customers. It allows you to accurately set the timing of cross-selling campaigns by predicting events in your customers’ life cycle and improve risk scoring by taking probable future behaviour into account.
It is very challenging to manage various technologies, methodologies and complex analytical processes effectively. Here is just a shortlist of hints based on our extensive experience with Data Science at Profinit:
- Always use a variety of modern yet proved tools. Do not focus solely on a single technology, and keep a spectrum of opportunities open.
- Have purple teams to benefit from the mix of competencies in business, statistics, data science, data engineering and programming.
- Add a time-series dimension to your models. Discover how to effectively use the information on how your customers use their accounts and other products. Use modern approaches to RFM analysis.
- Do not simplify! As inputs are becoming more complex, so are the outputs. You are no longer dealing with just a few customer segments. Now you can identify dozens of various behavioural groups.
Data Science Research
If you want to stay ahead, you will need to be a leader in applied research. That might sound like an exaggeration, but it is a fact. How can you beat the competition using technologies and approaches that are standard in the field? Your Data Science department has to be actively researching and absorbing new ideas. Cooperate with academics and make use of data science consulting services.
However, be picky! Always consider each idea and whether it applies to your business. Evaluate if you can get quick wins or any strategic advantage.
Nowadays, a lot of research is going on in the field of big data for customer micro-segmentation. Thanks to the high-speed processing of huge amounts of data, it is possible to dig into the deepest bits of information. The possibilities of transaction processing have reached such a level that billions of transactions can be explored within minutes or seconds.
Profinit is currently applying the results of its many years of research to constructing pseudo-social networks by combining transaction data of customers of various types. Our approach offers a completely new level of detail. It enables real micro-segmentation of customers.
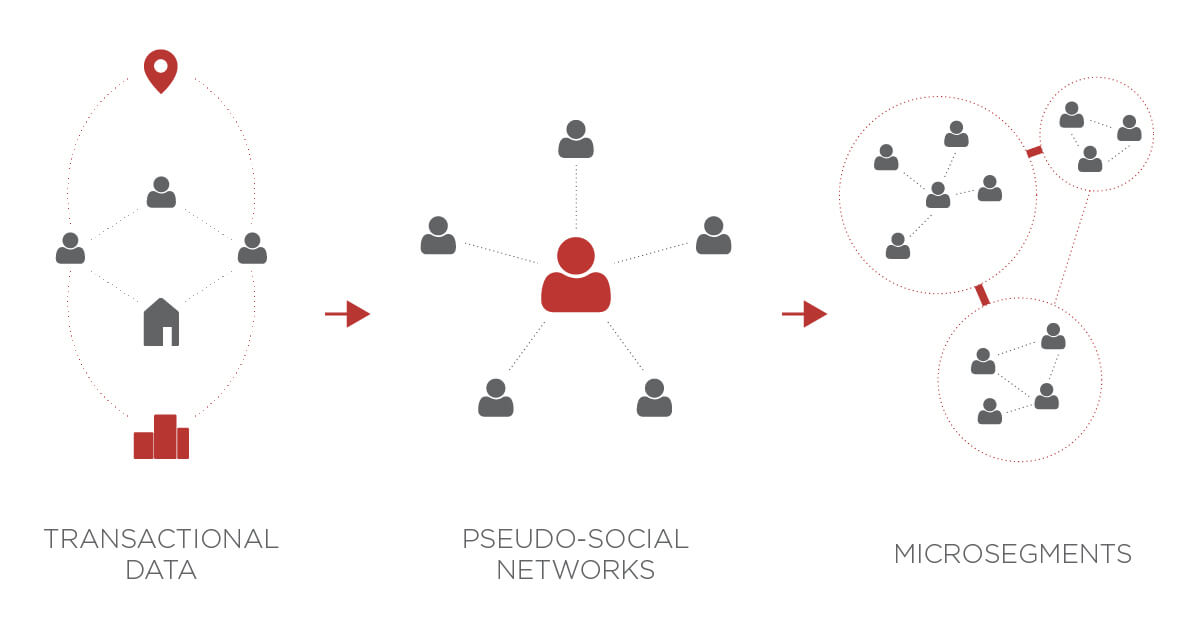
We are able to create a small behavioural bubble around each customer. Then we connect similar bubbles into chunks, let’s say micro-segments. This enables us to derive advanced similarity metrics for risk or marketing purposes.
– PETR PASCENKO, Head of Data Science in Profinit
Whichever evolutionary step you are about to take, always remember the essential purpose of your segmentation strategy. Customer segments are not valuable unless they are part of a business case.
As part of Profinit’s banking solutions, we use our unique approach to micro-segmentation to enhance the accuracy of its in-built models. Those detectors are ready to provide solutions for various domains such as risk or marketing. Learn more about how to discover relationships among your clients, their employers, their wallet-share and how likely they are to be interested in product offers.