How can banks increase loan uptake among their client base? (Hint: the answer is data-driven.)
To offer the right loan products at the right time, you need to understand how customers behave.
Increasingly, technology-driven banks are turning to machine-learning algorithms to make better decisions. When it comes to consumer lending, behavioural modelling is an effective tool that banks can leverage to predict loan acceptance.
But before we explore how this approach can be used to deliver keener insights, let’s look at a recent trend in consumer credit that’s raised the stakes for lenders.
Market hesitancy has provided banks with a wake-up call
Consumer lending has been one of the most profitable revenue channels for financial institutions in recent years. However, uncertainty caused by the COVID-19 pandemic has created caution among borrowers. According to recent ECB statistics, the volume of consumer credit has reduced.
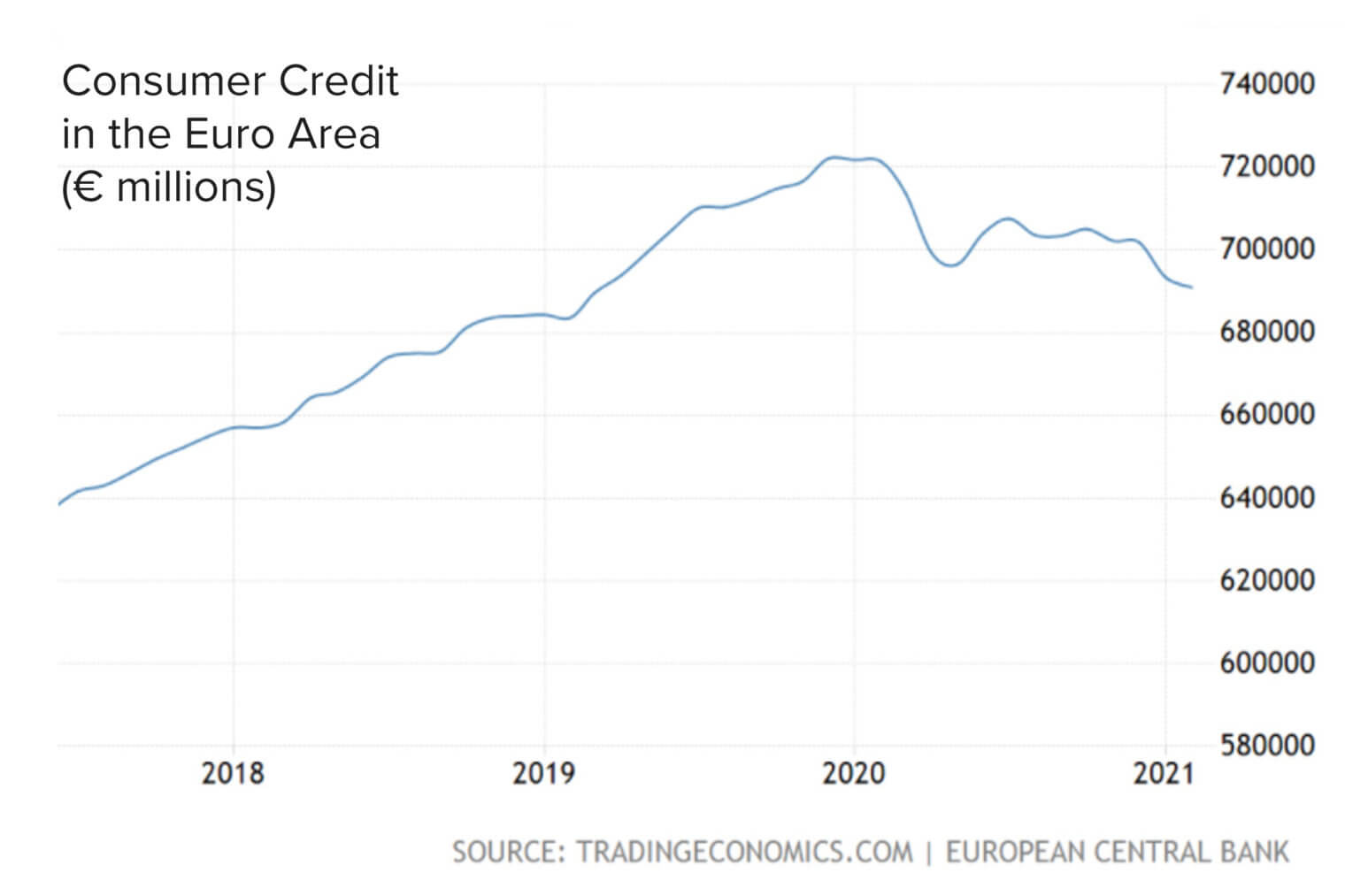
This downward trend has placed even more pressure on banks struggling to extract value from their loan portfolios. Fortunately, advances in data intelligence have thrown banks a lifeline.
Data-driven campaigns deliver greater value
The main reason campaigns have difficulty selling loans is because of ineffective targeting. The key to increase accuracy is to identify which customers are most likely to accept loan offers.
For lending purposes, we typically divide customers into the following 3 segments:
- Savers and less active customers who usually avoid taking out loans (~25% of the client base)
- Regular borrowers who take out loans all the time and need little encouragement (<5% of the client base)
- Receptive existing and new customers who often remain undecided (~70% of the client base)
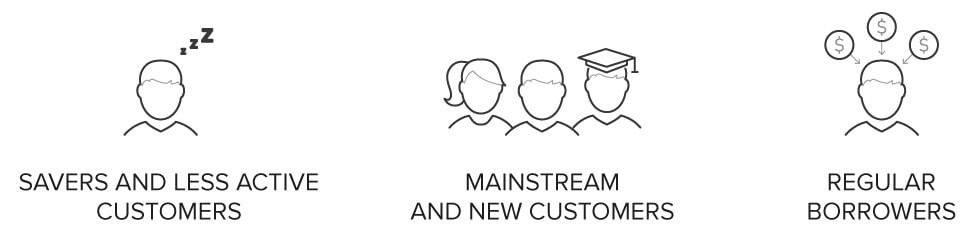
So which segment do we target? Well, all the low-hanging fruit has been picked, so we can rule out savers and borrowers. That leaves only one segment that’s ripe for conversion. To uncover value, you need to target that 70% majority made up of new acquisitions and existing customers. The ones who tend to “sit on the fence”. So how do you determine which of the individuals within this segment are most likely to take out loans?
Micro-segmentation provides those all-important details
Identifying your target group is all well and good. But that alone won’t boost your response rate. To do that, you need to zone in on the individual. In other words, a “segment of one”. This is called micro-segmentation. And it’s what takes campaign targeting to the next level.
Whether you’re offering a mortgage, credit card or personal loan, identifying the distinct habits and preferences that make your customer tick is what really matters. The more complete the profile, the more specific your messaging can be. As we’ll discover next, micro-segmentation is a key element in one type of predictive modelling.
To predict the future, you need to understand the past
Predictive modelling is about summarising the knowledge you’ve accumulated to date and applying that information to assess future events.
One tool that’s particularly useful for campaigns that want to acquire prospective borrowers is behavioural modelling. It’s a bit of a mouthful, so let’s break it down.
What do we mean by propensity? It’s basically a word that describes how disposed someone is to behaving in a certain way. Of course, the behaviour in question here is buying or purchasing. Modelling removes the guesswork by leveraging previous spending habits to predict future purchasing activity. Now that we understand the term, let’s look at how it works.
Augmented campaign targeting depends on a strong behavioural model
Traditionally, banks have always used transactional and socio-demographic data for risk assessment and credit scoring.
But propensity-to-buy is a more dynamic form of modelling. It factors in behavioural data from a wider range of sources to provide a more nuanced understanding of customer habits and preferences. These fused insights are then used to create a propensity score for each customer. Campaigns can then use this information to target the right people with the right offer at the right time.
Behavioural modelling in action
So what happens when we apply propensity-to-buy modelling to our majority segment of potential borrowers? As you can see below, the results speak for themselves.
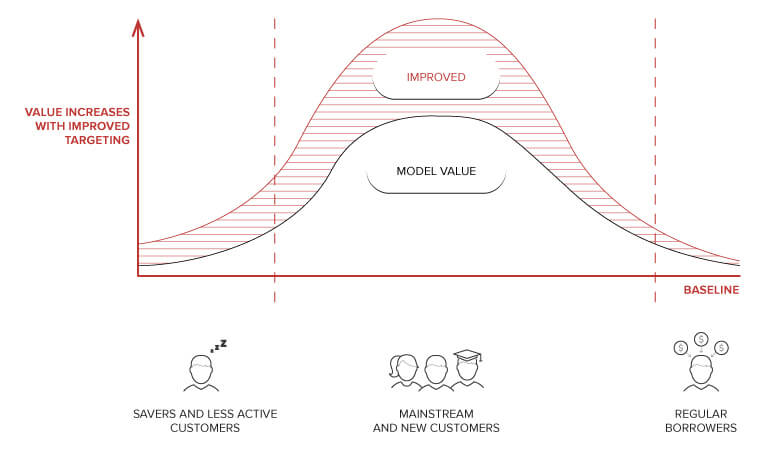
Successful targeting is about individuals, not customers
Let’s recap on what we’ve learned. The trend toward personalisation in the banking sector has transformed the way in which lenders interact with borrowers. Customers aren’t being treated as numbers anymore. After all, creating a mutually profitable relationship is one of the ways to increase a key metric for all banks: customer lifetime value.
But to really build engagement, assessing the acquisition potential of your current client base is essential. To do that, you need to:
- Know which customer segment to target
- Focus on individuals within that segment
- Use behavioural modelling to identify future purchases
Customer-centric modelling takes into account transactional, demographic and socio-economic behaviours. Adopting micro-segmentation and CX-geared initiatives are two important pieces of the targeting puzzle.
Can you ever 100% predict whether a customer will take out a loan? The answer is no. But what if you were able to determine loan acceptance in 87% of cases? Discover how Profinit’s propensity-to-buy solution mines data intelligence for targeted growth.