Data-driven incident handling
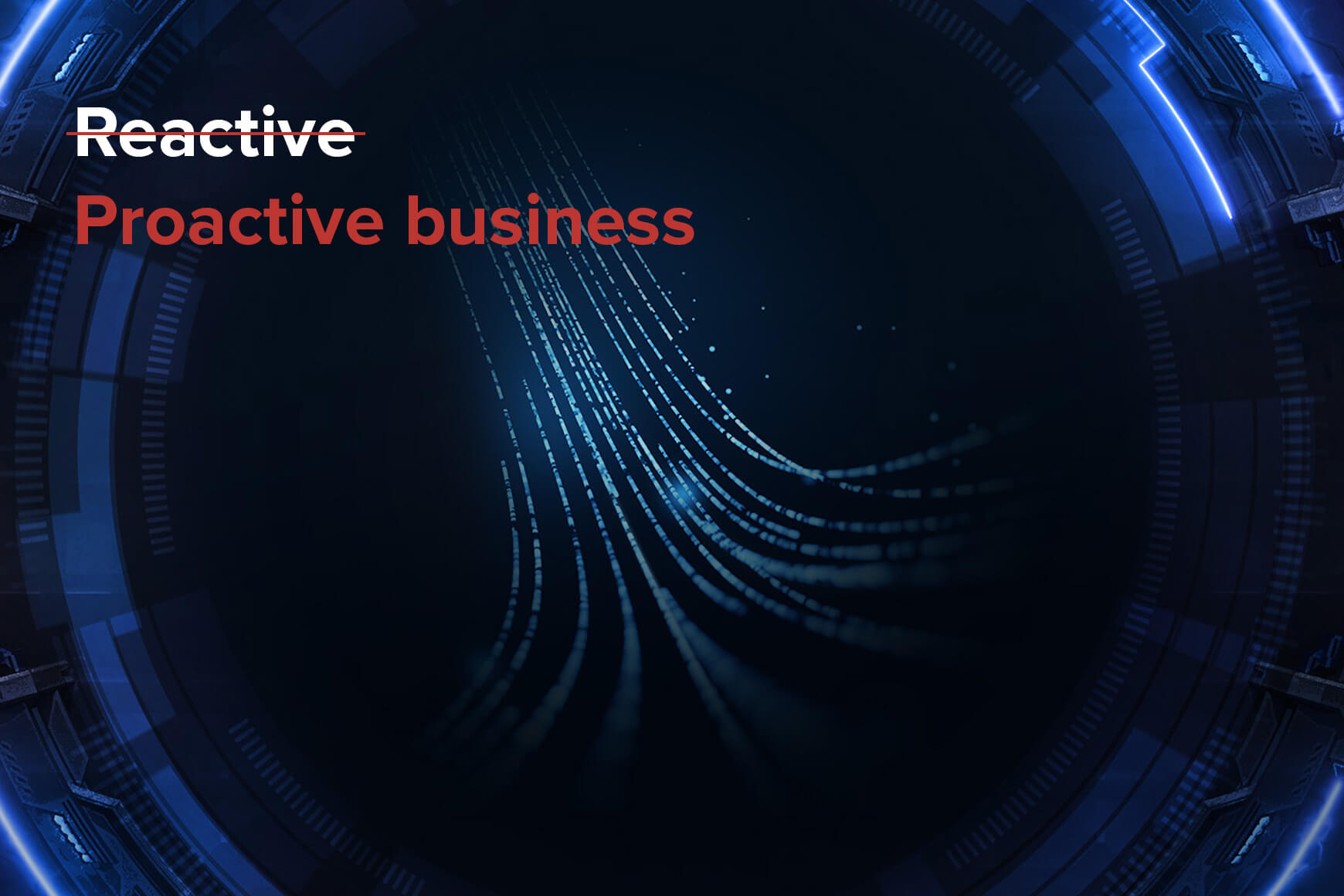
How to use data to shift your business from reactive to proactive
It is beyond any doubt that financial organizations have to focus on improving their processes for handling incidents. Whether we are talking about fraud, risk management or regular operations, every slowdown in incident-handling processes always cost dearly.
On top of that, slow processes involving clients introduce additional hazards. Apart from the direct losses caused by inefficiency in day-to-day business, they might have even worse indirect impacts. Losing business opportunities, diminishing client interest and worsening customer experience might also lead to reputational damage and customer churn.
Every slowdown in incident handling processes always cost dearly
Both investing in improvements and the automation of incident handling are beneficial and crucial for efficiency and building client trust. This article shows how to use data both to improve your processes and to completely shift from a reactive to a proactive approach.
Slow reactive processes of the pre-digital era
Let’s demonstrate the progress possible starting with a typical case of slow reactive processing. Imagine a scenario where a customer of an old-fashioned bank wants to resolve an incident involving a transaction that was not processed correctly.
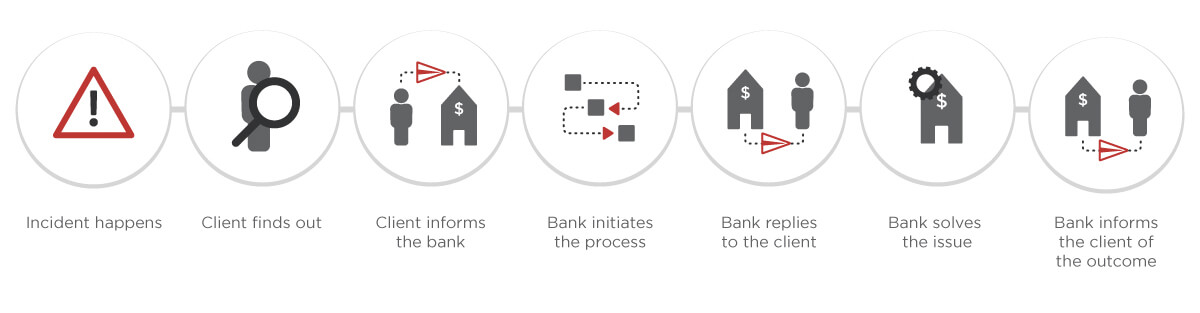
We are not discussing how long it takes or the quality of the bank’s response to the issue. The point here is to remark how much time passes from the moment the incident happens until the bank even starts solving the issue.
During that time, the client cannot feel at peace until someone at least replies to say they are working on the issue. The slow reaction time dramatically worsens the customer experience and increases the possibility of churn due to the loss of trust.
Even though modern times call for the digital transformation of the banking sector, it is still common to find such incident-handling relics among contemporary banking processes.
Reacting faster to the client
Every modern bank applies digital technologies to support incident handling. Various internet and mobile applications, chatbots and bug trackers make it easier for the customer to report the incident and receive a prompt answer. The customer experience is improved, the client is more involved and overall client-care is better.
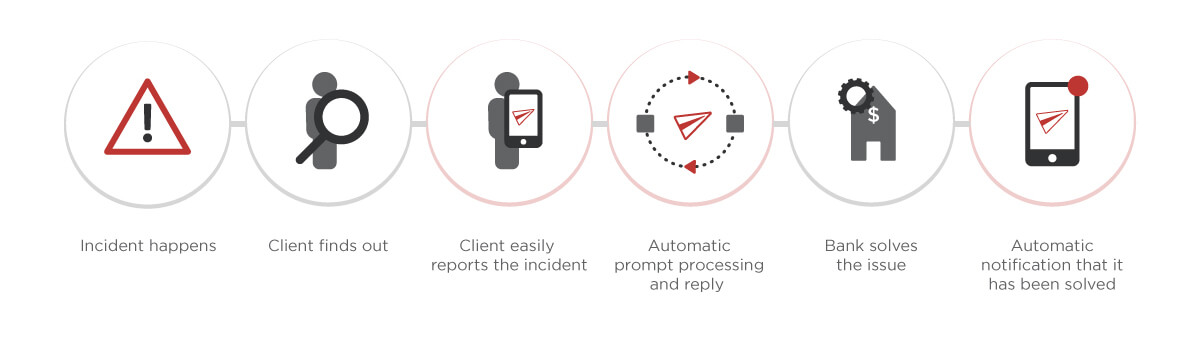
Clients are more comfortable with incident processing and communication. However, notice that there are still many steps between when the incident happens and when the bank finally starts solving the issue.
React as it happens by detecting incidents in real-time
How can you close the gap between the incident and its resolution? The answer is that it’s necessary to start detecting incidents in real-time. Here comes the first step in data-driven proactivity as it is no longer necessary to wait for clients to notify you about issues; instead, you can find out what is wrong based on data monitoring and collection done by operational banking systems.
If issue processing is automatically initiated and follows real-time detection, then you actually start solving the issue as soon as it happens. Notice that the process is initiated at the same time (or sometimes even before) the client finds out about the incident. This means you can take the initiative to notify the client the proper way so that the client won’t be surprised and you can assure them that everything will be handled smoothly.
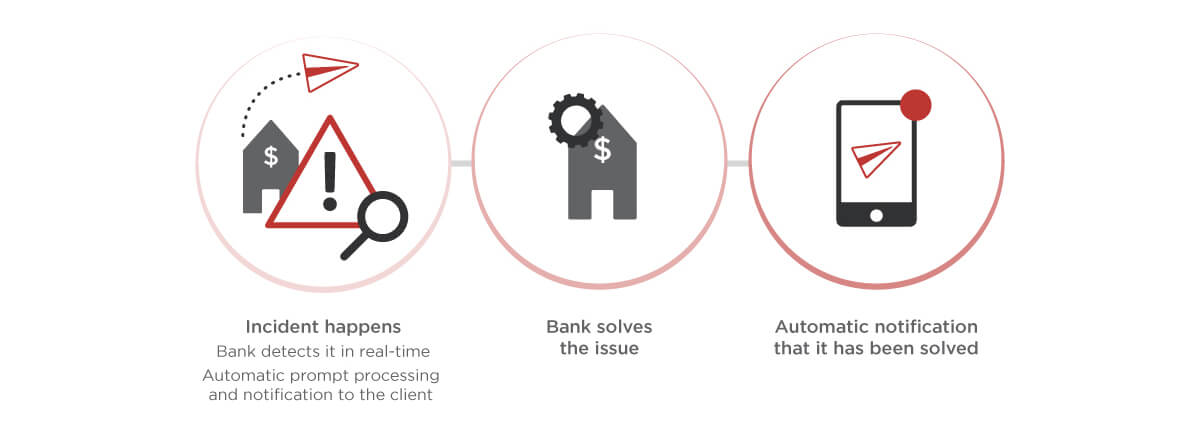
Just don’t forget one important thing. Even if the incident detection and communication with the client are handled perfectly, it is still necessary to quickly and successfully solve the original issue. Otherwise, the client will still have a bad experience and the risk of losing their trust and potential churn is still present.
The data-driven proactive approach
Start solving the issue even before it happens.
Here we come to the final point, revealing how to improve incident handling even more. The answer lies in even better use of data and predictive analytics. Stop being reactive and act proactively. Become utterly data-driven. Adopting this approach, you can start solving issues even before they happen. Using predictive analytics to prevent incidents not only saves money on fixing issues but also on issue processing and potential impacts on client satisfaction (churn, communication, client aftercare, reputation re-establishment, etc.).
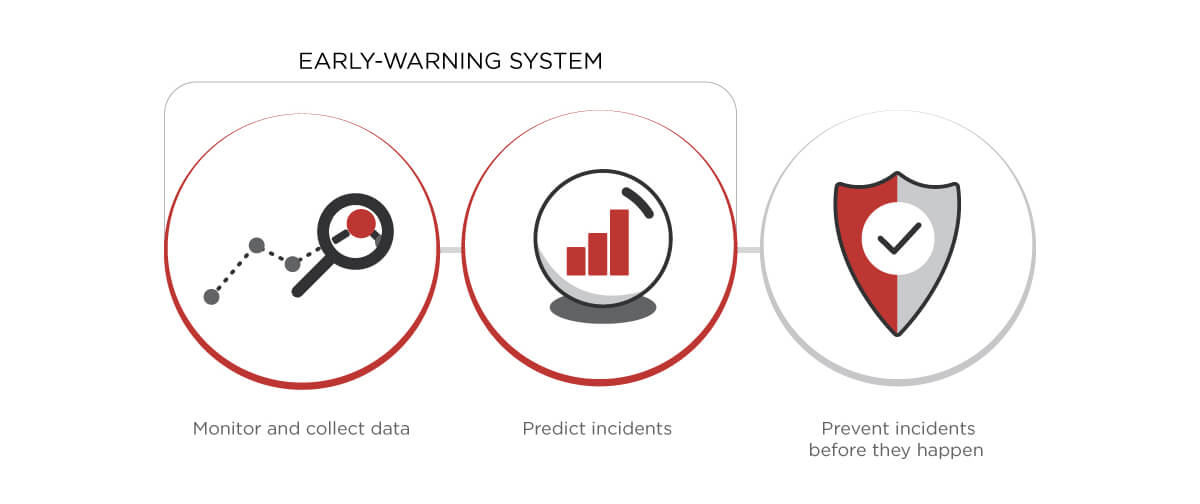
As a part of our predictive data analytics for banking, we often apply the so-called early-warning system, which is a proactive solution that predicts incidents and automatically prevents possible threats.
The proactive approach to credit risk
The proactive approach described above does not apply only to operational incidents. An excellent example is predicting credit-risk change using customer behaviour analysis to prevent defaults.
Even though a client may successfully make it through credit scoring and obtain a loan, that doesn’t mean their situation can’t change later on. After some time, the danger of default might increase if certain events occur.
- Job change/loss
- Income shortage
- Higher expenditures
- New loans taken (e.g., provided by non-banking organizations)
- Change in household situation
- Change in social environment
If such an event occurs, it doesn’t mean it will certainly be a problem right away. Nevertheless, it might have an effect in the future.
A red flag is raised once the predicted risk of default gets higher as negative behavioural changes accumulate.
In case of using the reactive approach, the bank will understand the problem when a payment is late or when the client comes into a branch. By then, it is usually late in the game and the risk of default is already very high.
On the other hand, the proactive approach using the early-warning system would monitor the situation from the very beginning. It is a data-driven process that collects and processes data about the customer from transactional history, computes a social score, etc.
The point here is to detect changes in time and proactively predict the possibility of default. In such a case, a red flag is raised once the predicted risk of default gets higher as negative behavioural changes accumulate.
The bank can know about the problem even before the client realizes that something is going wrong and help him prevent potential threats in time. This is very important in building trust and solid relationships with clients.
Conclusion
Here is a summary of how to approach incident handling in order to increase efficiency, prevent churn and negative customer experience and increase the trust of your customers.
- Collect data. Be data-driven.
- Detect incidents as they happen. It dramatically decreases the period between incident occurrence and resolution.
- Monitor and make predictions based on the data and prevent future incidents. Avoid incidents entirely.
- Proactivity will enable you to take the initiative and create business opportunities.
Related topics: 4 most important applications of data science in banking