Book Review: The Book of Why – J. Pearl
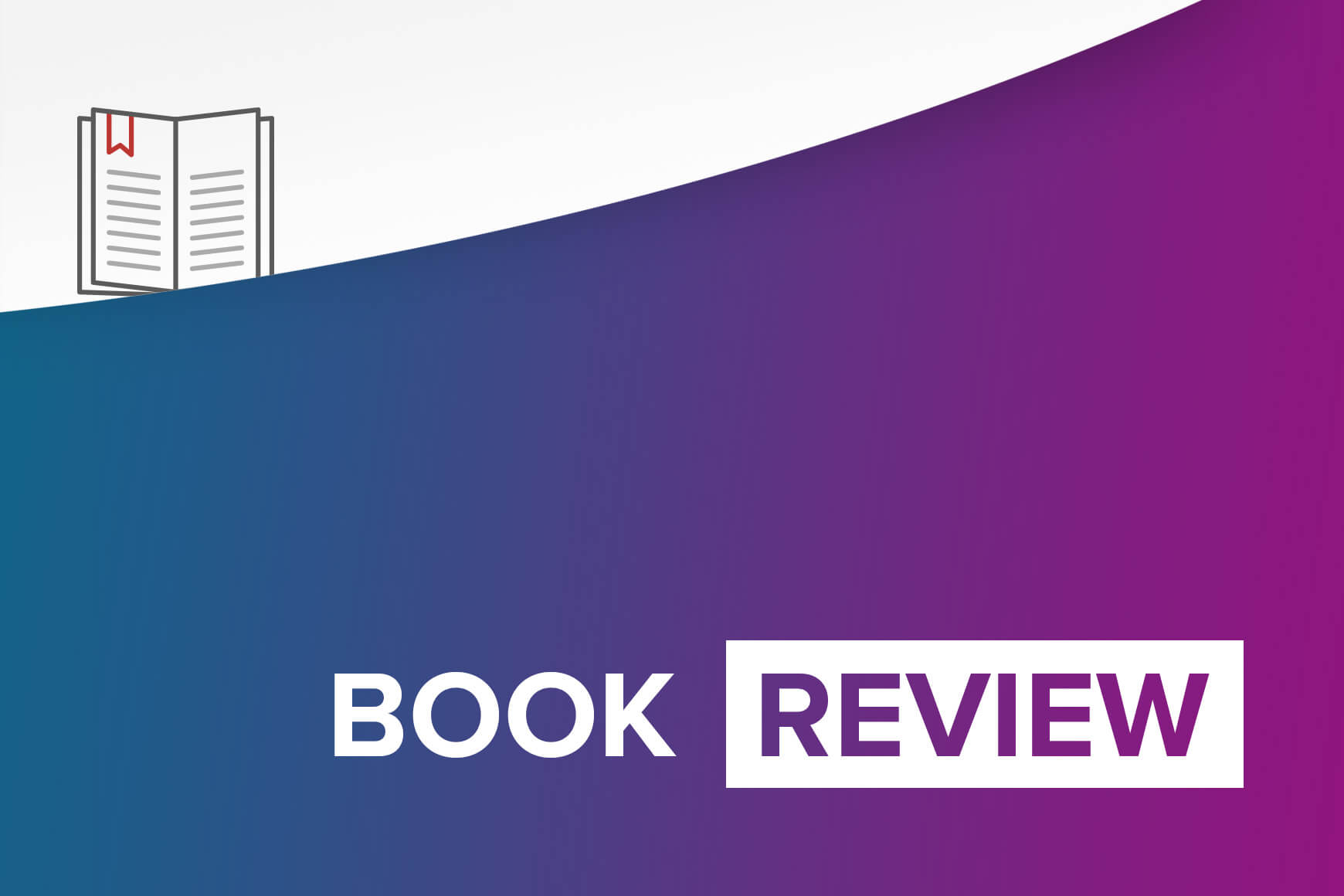
Why the why? As a statistician or data scientist, I want to answer many why questions.
• Why are these customers buying the product?
• Why are these patients not getting better?
• Why is the number of defaults rising?
• Why…
And it seems there are many tools in my data scientist toolbox to help me find the right answers. Or are there?
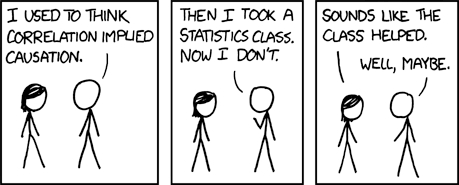
Right, every statistics student has had it drilled into his or her head: “Correlation doesn’t imply causation.” However, to answer the why question implies detecting causation.
Lack of statistical causation tools
Does the sunrise make a rooster crow, or is it the rooster that brings in the morning? This is a trivial question for a human being but a hard one for a statistician. It is impossible to answer it just from the data itself. I can find a dependency, I can prove it and quantify it, but I cannot say which causes which.
For centuries, randomised controlled trials (RCT) were the only way to prove causation. And, as they cannot be applied to observational data, they can be expensive, slow, or even unethical.
Smoking-cancer debate
One of the examples in the book concerns the smoking-cancer debate, which started in the first half of the 20th century. Imagine asking test subjects (that means people) to smoke in order to prove that it would kill them. Or worse, telling smokers that they are not allowed to smoke! This is highly unethical, not easily controllable, and very lengthy. As a result, RTC were of little use and the necessary tools for proving causality in the observational data were non-existent.
For this reason, the smoking-cancer debate took a few decades. It was clear quite early that the connection between smoking and lung cancer was there, but that was just correlation, not necessarily causation. Let’s look at some causal graphs.
We want to prove:
But what if there is a common cause for both smoking and lung cancer, for example, a genetic predisposition that makes people more likely to smoke and causes cancer? If you have this predisposition, no matter whether you smoke or not, you are still at a higher risk of developing lung cancer. The smoking and cancer would be correlated but without one causing the other.
The Book of Why tells this story like a detective novel (the evil statisticians outlawing the term causality against the heroes with the new approaches). Today we all know that smoking kills. Who can we thank for the proof? To find out, you have to read The Book of Why.
Do-calculus
The author does more than just expose the statistician’s blind spot. He offers a general solution: do-calculus. Using causal graphs like those above to make an additional assumption about the individual connections, do-calculus can prove causality even in observational data. And who knows, we might witness another barrier being surmounted here on the way towards a real thinking machine.
The book
The book reveals some limitations of current statistical methods using paradoxes and real historical examples. The author accuses several key statisticians (e.g., R.A. Fisher) of obstructing progress, which may divide readers, a bit like Black Swan by Nassim Taleb. Personally, as a statistician, I welcome the outside-the-box perspectives exposing these shortcomings, especially because solutions are proposed.
About the author
Judea Pearl is an Israeli-American computer scientist and philosopher, well known as a Bayesian network pioneer. He was awarded the Turing Award in 2011 for fundamental contributions to artificial intelligence through the development of a calculus for probabilistic and causal reasoning. Judea Pearl is still active at age 82, and you can even follow him on Twitter (@yudapearl).
Get in touch with us for a free Data Science consultation.