Book Review: ‘How not to be wrong’ by Jordan Ellenberg
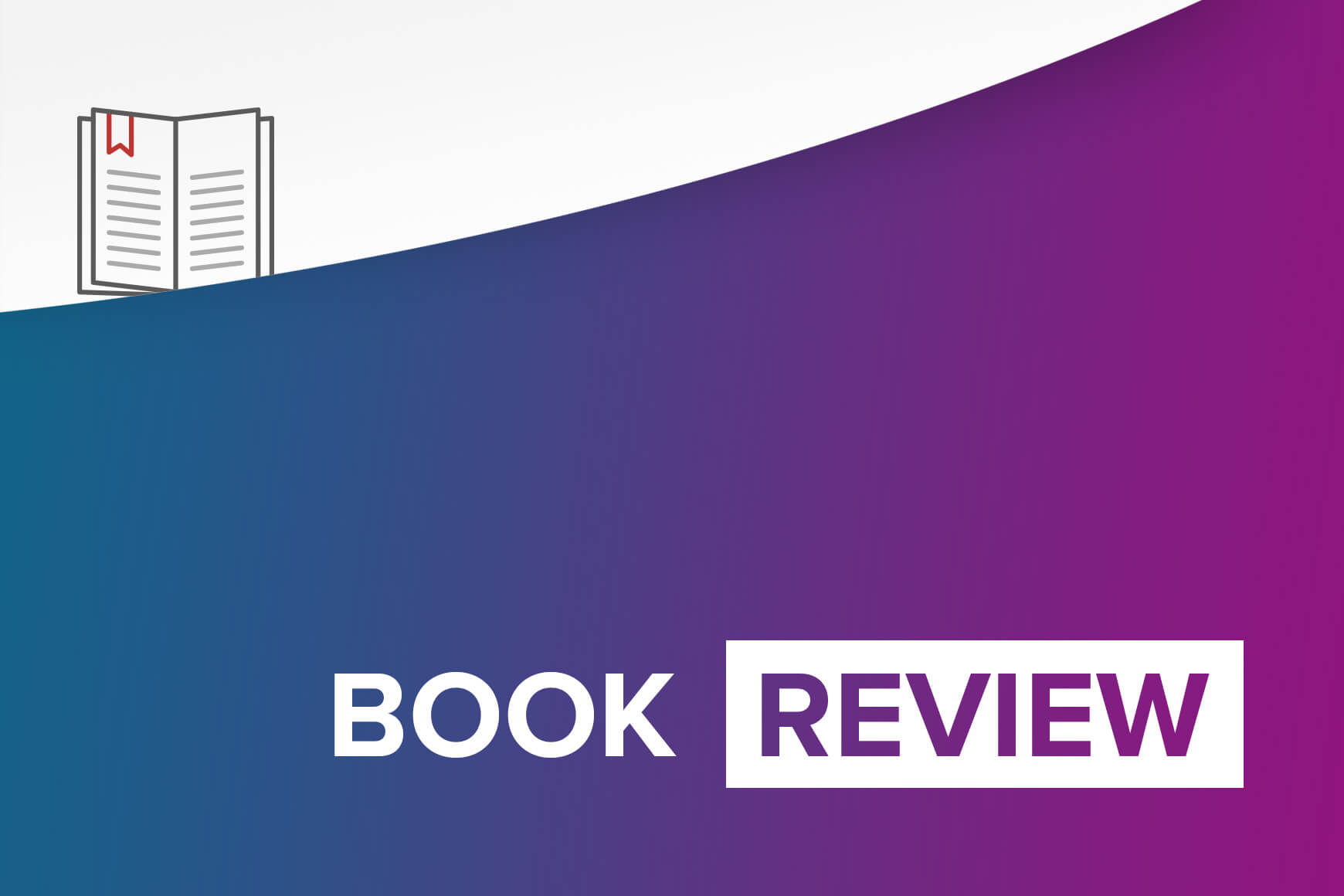
About the Author: Jordan Ellenberg is the John D. MacArthur Professor of Mathematics at the University of Wisconsin-Madison and a 2015 Guggenheim fellow. Author of a few books on number theory. He has written articles on mathematical topics in the New York Times, Washington Post, Wall Street Journal, and many others.
Why I liked this book: It’s fun to read about familiar concepts in the wild, how mathematicians tried to “solve and understand the life” and how they failed or succeeded.
What makes this book harder to read: There is no coherent storyline in this book. It’s a pack of different stories loosely coupled together with additional philosophical off-topics. As a result, you can read chapters in any order you like.
Style: Can’t say anything special. The book is fun and easy to read. I love that the xkcd comics found its way into the book as well.
_________________________________________________________________________
When I studied math at school, I felt like it’s way too abstract. What is the meaning of this specific equation? Will I ever need calculus in my life?
The book ‘How not to be wrong’ by Jordan Ellenberg explores a lot of mathematical topics in the context of real-life applications. It is full of personal stories from mathematicians. To a data scientist, the concepts explored in this book are old hat: integration, p-value, expected value, correlation, linear regression. Most students simply learn them by heart in a very boring way but fail to understand how they translate into real scenarios – because life happens outside the school. However, this book does a great job at explaining mathematical concepts through interesting stories; all without using a single formula! I believe that it is important for anyone working with data to sharpen their intuition about these concepts and that’s why I highly recommend reading: ‘How not to be wrong’.
Here are a few stories from the book to warm up your appetite:
P-hacking or how to torture the data until it tells the right story
P-value is a common tool for the evaluation of scientific experiments. But like any other tool, it is helpful for certain situations and really unsuitable for others. In some cases engineering, your p-value can be even dangerous. When misused it may, for example, prove that a dead salmon is pretty good at recognizing people’s emotions. [1]
This is, of course, an extreme example. Nevertheless, researchers ask all kinds of questions, and might sometimes receive positive results by sheer chance, although the proven effect does not exist. If you are a fan of the comic strip ‘xkcd’ like us, you know what I’m talking about:
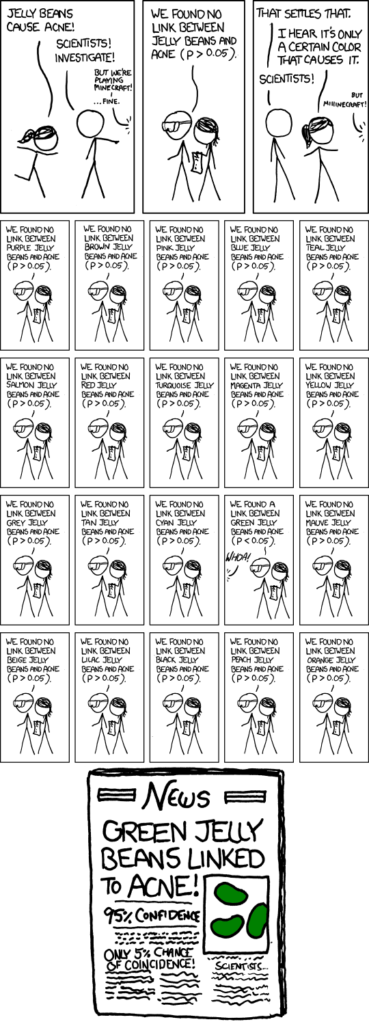
In the second chapter, Ellenberg mentions messages hidden in the Bible, Torah, and Dostoevsky’s books. You will learn how to calculate the probability that your neighbour is a terrorist. Or the probability that God exists (personally, I think it is 42 J). And if you don’t know it yet, what Bayesian inference is all about.
When to play the lottery
Another thrilling story from this book is built around the concept of expected value. What would happen if you designed a lottery with a positive expected value? That is, the lottery in which on average you win more than you spend on a single lottery ticket? Well, if you studied IT, you might want to buy as many lottery tickets as possible. Read about what happens next in chapter 3.
One math trick
It was always hard for me to think about high-dimensional data. We all can imagine a 2D plane and two-dimensional data. If we try a bit harder, we can imagine 3D planes. However, data in data science practice usually comes in multiple dimensions. What are you supposed to do with that? Maths says we should do nothing. From its perspective, there is nothing special about multidimensional spaces. Look at this example from the book: On the top, there is a 2D vector, and below there is a 10D vector.
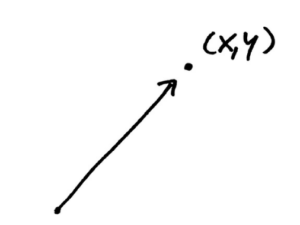
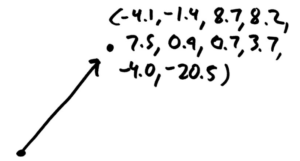
It almost feels like cheating, but it isn’t. In fact, you can carry out all your computations in any number of dimensions that you need and use 2D as your intuitive tool for what is going on. Maths is really beautiful…
Summary
I will stop spoiling right here. By now, you have already decided if this book is worth your time and money, or not. If you figured that it isn’t, I highly recommend watching this video, where the book’s author goes into more detail about the lottery story.
References:
[1] Neural correlates of interspecies perspective taking in the post-mortem Atlantic Salmon: