AI in Banking – 4 major pain points in the DACH region
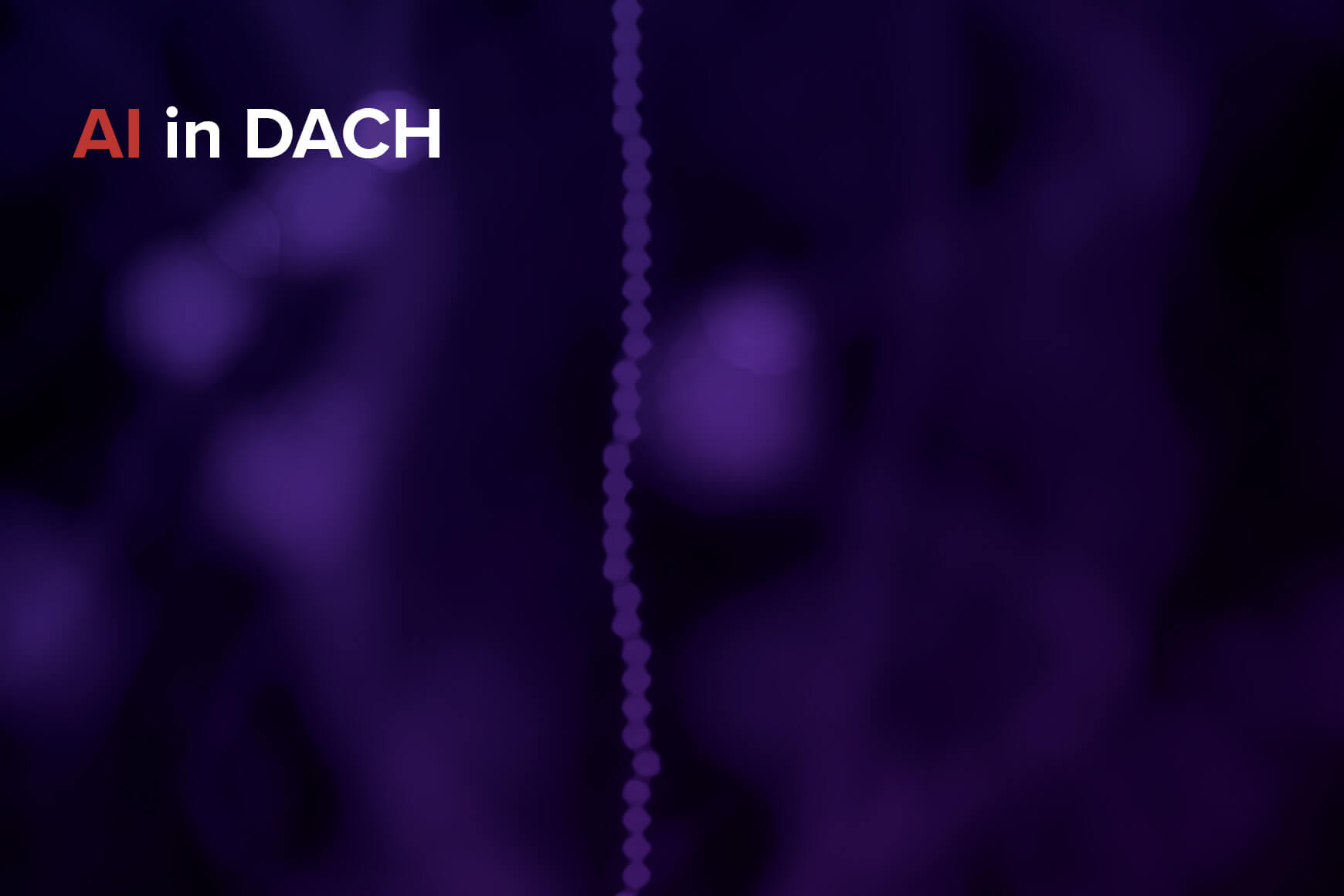
Despite the common thrashing German, Austrian, and Swiss banks often get at conferences relevant to the topic; they do put a significant amount of effort into adopting AI for meaningful use cases. However, as with all innovations, the financial industry is still dealing with some pain points concerning AI.
1. Digital Washing – Chatbots are no AI
Amongst the influx of buzzwords and the inherent need for cool branding, many banking institutions invested large sums in their own start-up hubs, spin-off Fintech companies, and digital products that are visible to the customers. However, there is a big difference between machine learning applications, such as chatbots and actual complex AI. To cite Mat Veloso:
Difference between machine learning and AI: If it is written in Python, it’s probably machine learning. If it is written in PowerPoint, it’s probably AI.
2. Customer-orientated vs. Profit-orientated
More than anything, this is an attitude issue. If you want to implement AI in your bank, start asking yourself why. If the answer isn’t: “Ultimately making my clients’ lives easier” – forget it. This is a problem we often face with our clients, who want to deploy sophisticated tools at all costs, forgetting the underlying motivation in the process. In many banks, especially if we compare traditional houses vs. challengers, this is a major problem in thinking and company culture. If you put a bunch of bankers in a room and ask them whether banks should be more customer or profit-orientated, you will get a very mixed response.
In addition to that, in the DACH region, we often see the tendency for people to define themselves through limitations as opposed to opportunities (this is often labelled as the ‘American mindset’). Historically, conservatism is deeply rooted in the banking DNA and its structures. Anyone, who has ever witnessed attempts of an Agile transformation in a bank knows, how difficult this change of mindset can be.
3. Human Resources
You will need the brightest brains in the bunch to come up with and successfully launch profitable AI use cases in a bank. What a modern AI engineer craves the most is unlimited computing power, access to big data sets, and technology stacks. While banks can provide this very well in theory, they still struggle to attract young talent due to the traditional reputation of the industry as stale and inflexible.
4. The Level of advancement
The differences in AI adoption, digital transformation & Co. could not be much larger in the current Central European Financial landscape. We have large banking houses, who responded to the call and are pouring incredible sums into building internal know-how in AI, ML, and Data Science (Commerzbank, Raiffeisenbank Int., Bank Austria, to name a few examples). At the same time, challenger banks and Fintechs are at the forefront of recent trends and developments, setting an example for excellent customer experience, KYC, etc.
In between, there remains a large cohort of institutions, who are caught up in two worlds (e.g., German Sparkassen). We see grandly presented attempts at transformation, which pay lip service to the buzzwords of Cloud, or Scrum, but remain the same in their core.
Solutions
We have been helping financial institutions, banks, and insurances with Digital Transformations, Legacy Systems, as well as Big Data & Data Science adoptions for over 20 years now. Get in touch with us to arrange a meeting and talk about your needs in this area!