The 4 most important applications of data science in banking
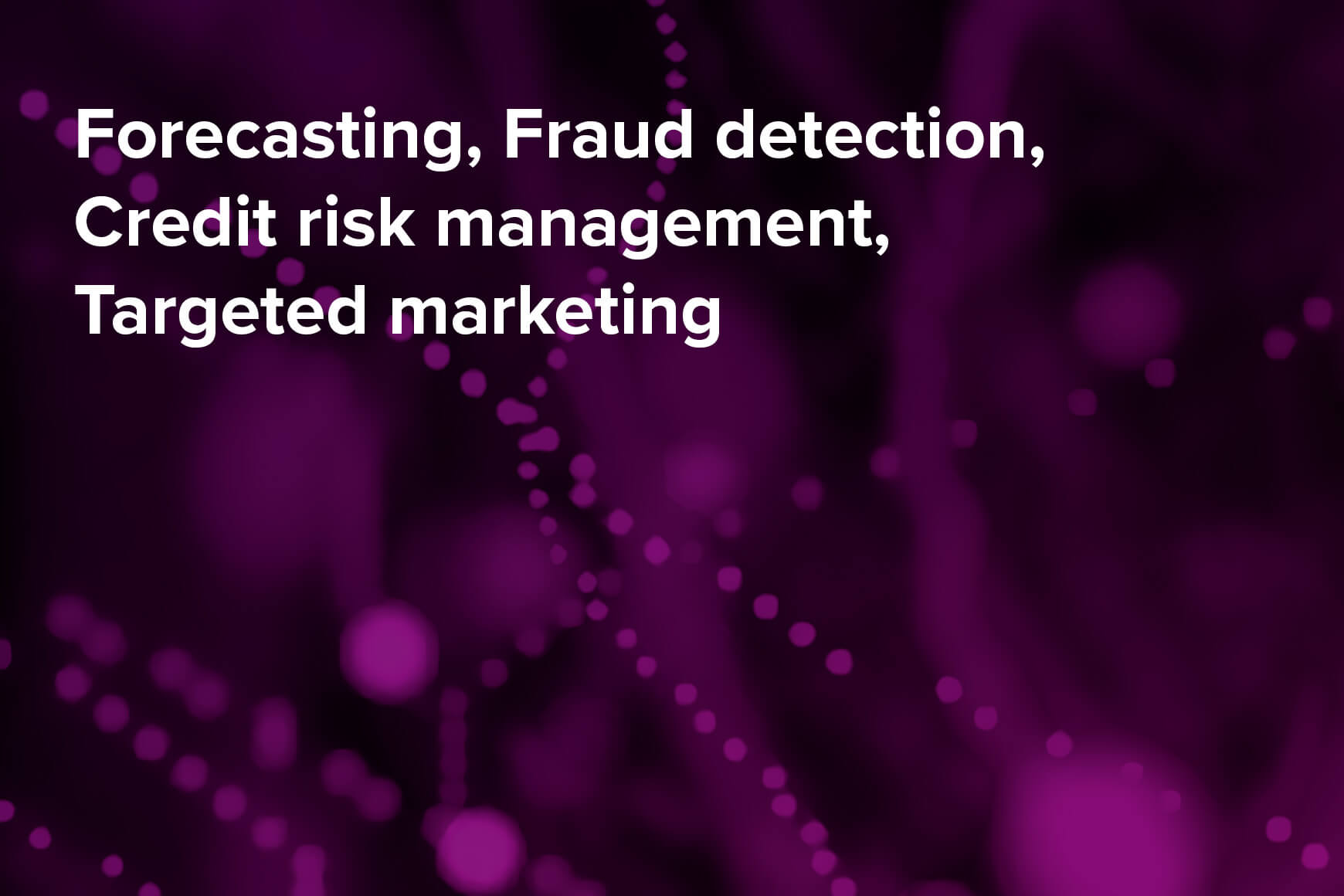
The Banking/Financial sector is just one of the many industries, which benefits hugely from data science-driven insights. With a natural affinity for numbers and quantitative methods, it surprises no one that it was one of the early adopters of Data Science, Machine Learning, and AI to generate more revenue. While applications are endless and the research questions will be different in every specific context and bank, four general themes matter most related to data science in banking:
1. Forecasting
Predictive analytics and forecasting have occupied a dedicated space in banking for a long time. However, with the advent of Machine Learning and Artificial Intelligence, the scope of things that can be forecasted has significantly widened. The accuracy of models is improved, and the level of human intervention required slowly dwindling. Most essential applications concern, for example, revenue forecasting, or stock price predictions.
In reality, most banks have specialized departments solemnly focused on predictive analytics, which can (but will often not) identify as Data Science divisions. The actual labelling can vary from bank to bank.
2. Fraud detection
Fraud detection is a big topic in banking. A team of good data scientists will be able to flag suspicious transactions in a bank’s client data and build models that can determine the probability of activity being fraudulent with high confidence.
Most of the time, fraud detection is dealt with as a classification problem (this article offers a good, yet concise insight).
3. Credit risk management
Credit scores are the alpha and omega of mortgages and loans. Banks depend on understanding clients’ risk scores and financial behaviour for their business. Data scientists use existing data, such as details about past loans and defaults to assess the risk of a new client.
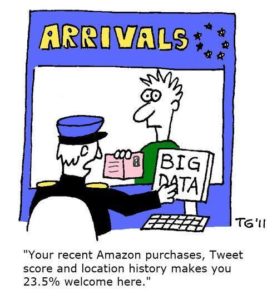
4. Targeted marketing
Data Science offers a powerful tool for customer segmentation and as a result knowledge about how banking clients are different/similar to each other and how they cluster. It goes without explaining that the better a bank understands its customers, their demographics, and needs, the better it can market its products through targeted marketing and individualized offers.
At Profinit, we have developed and deployed great algorithms to pinpoint various information of interest about clients, that some of Europe’s largest banks are currently using to win over more customers.
To understand data in the Banking sector and use it well for business, it doesn’t just take a good data scientist; it takes a damn good data scientist. But as we all know, these can hard to come by.
Why not working with us? Our team of 60 top-notch data scientists experienced in the Banking & Finance sector can help you to get the most from Big data and Data Science using state-of-art approaches. Get in touch for a free consultation!