How to Succeed in Data Science Projects
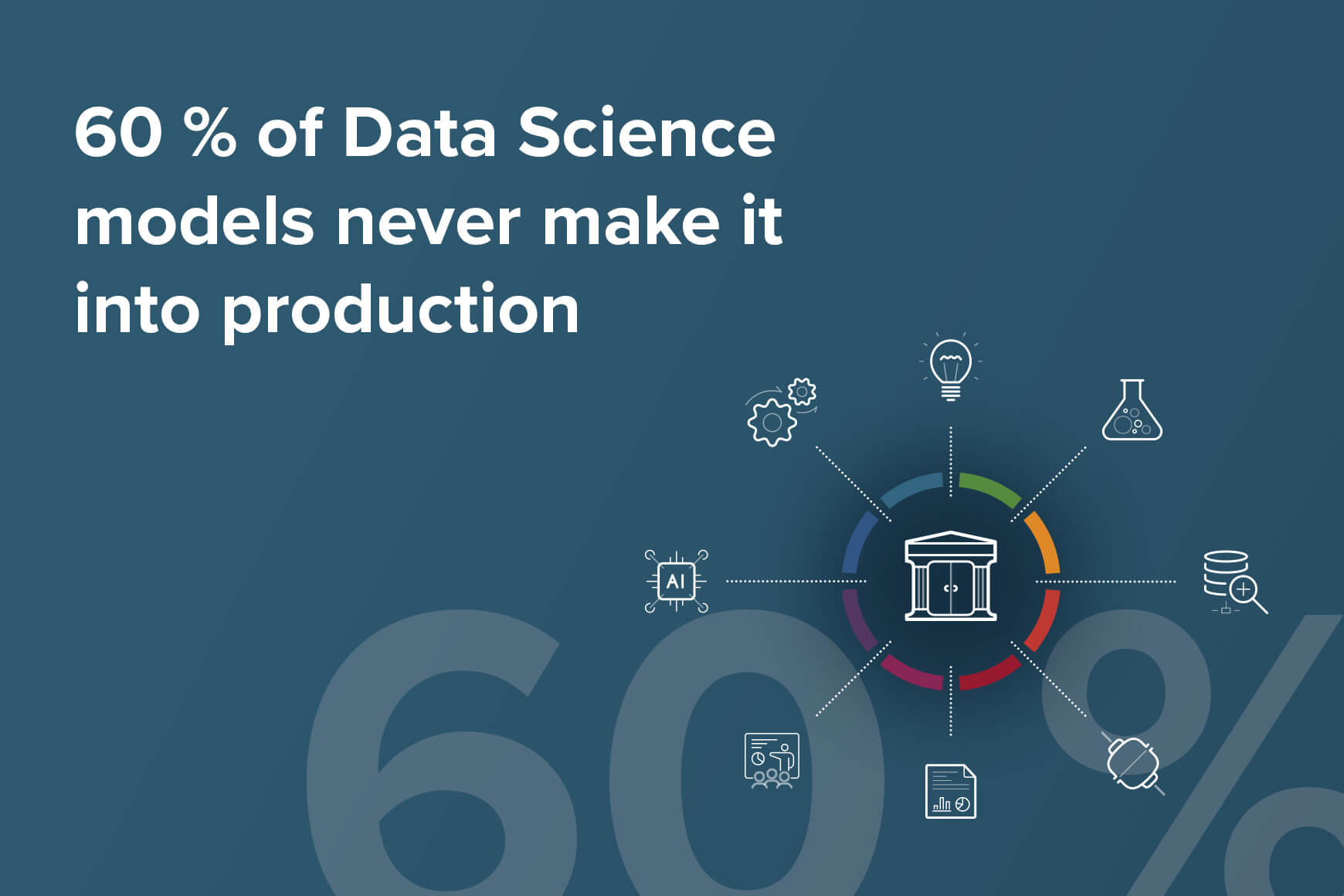
The effectiveness of data science projects is one of the hottest topics in business intelligence nowadays. On the one hand, we are discussing the huge potential of artificial intelligence in executing sophisticated tasks for businesses. On the other hand, we see the harsh reality of today. Large companies like banking institutions are struggling hard to deploy advanced data solutions and incorporate them into their not always up-to-date infrastructures. According to Gartner, 75% of data collected by businesses remains unused, and more than half of the data analytics models never make it to production. What are the reasons for such inefficiency?
BUILDING ANALYTICAL COMPETENCY
It is beyond any doubt how well companies understand the importance of building their data competencies, especially in such fields as banking and insurance. Data are valuable, and it is necessary to understand them and be able to use them to the fullest extent. The ability of the internal data analytical departments to understand business topics has to be continuously groomed and developed. The business know-how has to stay in-house.
Therefore, every modern data-driven company has key initiatives to build data science or analytical assets. This means building and maintaining teams with a wide range of knowledge and technological skills. Such procedures implicate finding and hiring highly specialized people (mostly with a strong mathematical, statistical, and technical background) and making them understand the complexness of the business domain.
Building such complex and wide-spread competency is an arduous and lengthy process. Even just finding and recruiting people with sufficient skills and backgrounds can be extremely difficult. And it is even harder to develop the competency to successfully achieve the desired quality.
RESEARCH VS BUSINESS
Turning talented, but usually, business-unaware, people into domain specialists are an integral part of the research and development in every industry. In practice, the result of such a change is the transformation of raw data research into fully-operational process know-how. Yes, we are talking about research and development here (and its first real contact with banking after a very long hiatus).
It is vital to understand that the basic idea of research is to discover what is working and what is not. In line with this perspective, the numbers at the beginning of the article don’t actually look so terrible. In the field of academic research, even applied research, having forty per cent of solutions end up usable is quite a high number.
On the other hand, business requires planning to measure success and profitability. It naturally demands that data science and analytics not be left only as research activities. Data science in production has to be managed and planned just like any other project. From the project management point of view, it is all just a matter of risk.
DATA SCIENCE PROJECTS
Executing projects in a low data-science-maturity environment means high risk for most, even for simple basic tasks. It not only depends on the maturity of the team members but also on how much research has been done, how many dead-ends have been discovered, and how much of the data have already been correctly understood.
Planning the right data science projects is like picking cherries! And executing them is never a routine job. If it were, then we wouldn’t be talking about research projects that bring you new insights and competitive advantage, but rather about applications of known technologies with expected outcomes, which is never the case. The fact that data science projects are not routine does not mean that they are without any order. Using the right research methodology, gaining the right experience, and achieving suitable data understanding maturity reduce the risks and help identify the wrong course right from the beginning.
THE ROAD TO SUCCESS
As discussed above, it is crucial to build your own data science competency. To do this, expert knowledge and the precise adoption of a proper methodology are necessary. Then the risk of your research activities ending up in a vicious circle decreases, and you can focus on generating new insights and desired solutions for your business.
- What is essential on the road to success is choosing the right technology stack that will serve you well but let you stay independent.
- Stay goal-driven, set up criteria, and measure success.
- Use an iterative approach, expanding from small MVPs to larger scopes. This attitude enables you to pick the right cherries.
- Always consider the risks when planning and executing. It is important to use your internal competency, but be ready to get outside experience if necessary.
- Use proven solutions and successful concepts. Be able to integrate them into your infrastructure and enhance your data competence.
- Bring in experienced consultants with the necessary knowledge and technological background.
Trusted external suppliers might help you with risk sharing, as they can provide experience with a similar problem, client, or industry, using proven methodologies and technologies. They usually focus on executing tasks that are similar in nature, which makes them proficient. Their business is to do repeatable and reusable procedures faster and more accurately. That’s why they can carry more substantial risks and bring added value by enhancing the experience of your team.