Building a Data Science and Analytics Competence in Banking
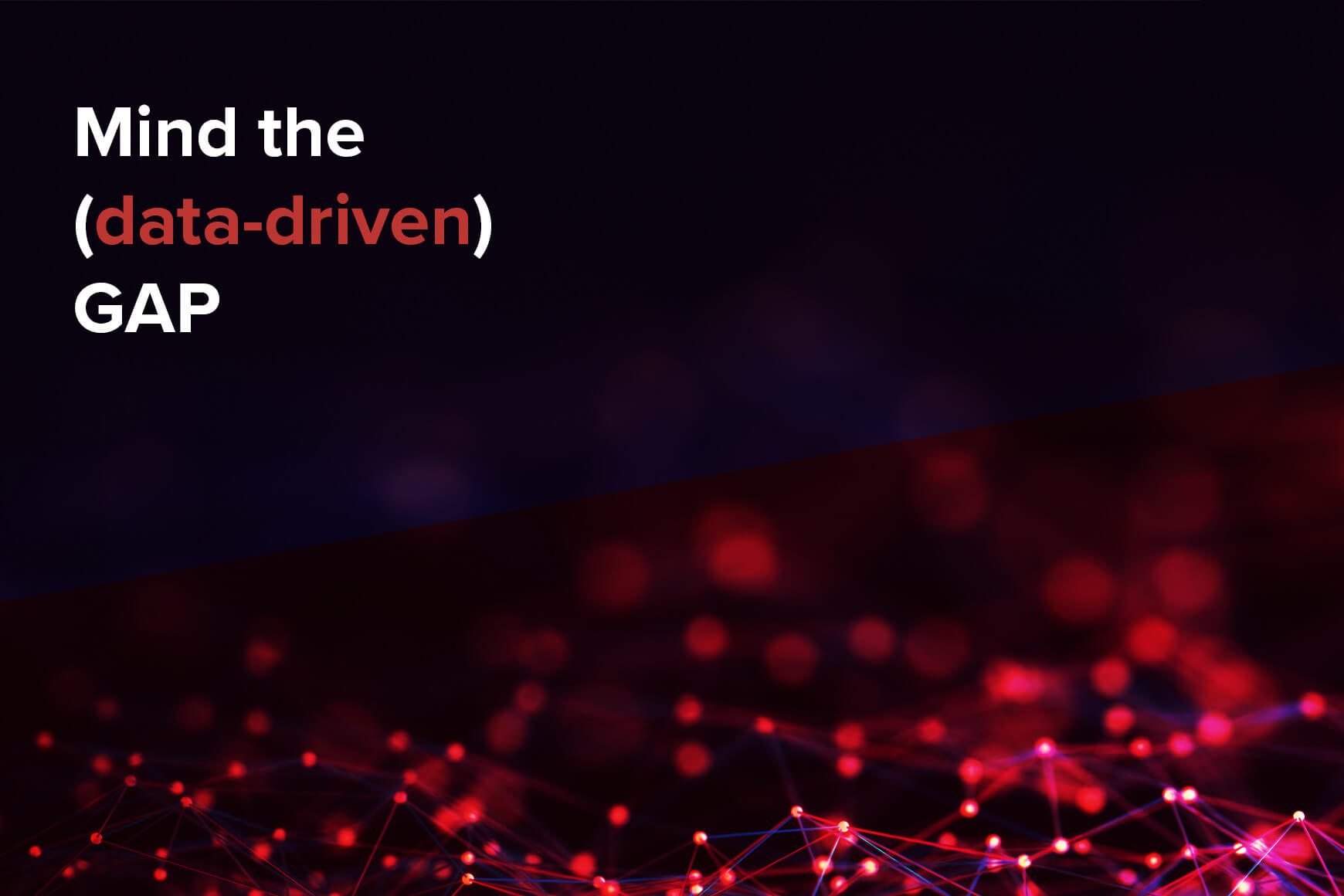
In the last five years of providing data science as a service to Europe’s largest banks, we have faced plenty of cases where banks were unprepared to work with their data.
Unfortunately, this problem arises not only at the stage of creating a competency in the field of data science and analytics, but also when banks are already data-oriented.
Here are three important observations we have made so far concerning the European banking sphere.
1. The widening gap of being data-driven
As mentioned above, we have been working with companies around the world for five years. Our data science portfolio includes cases spanning from the maintenance of a nuclear reactor to the optimisation of insurance policies.
Yet, the case of data science in banking is a very particular one. The level of application typically falls on a scale. One might expect that most European banks would be at the upper end of the spectrum. However, in reality, the range between individual banks is much wider.
There are some significant innovators on the market, who are way ahead of everyone else. In most cases, these were the early adopters of the data science approach (e.g., Deutsch Bank, UBS). By all means, that has proven to be a smart move. With the introduction of PSD2, at the very latest, banking institutions were forced to use their data. Otherwise, a third party had to do it for them.
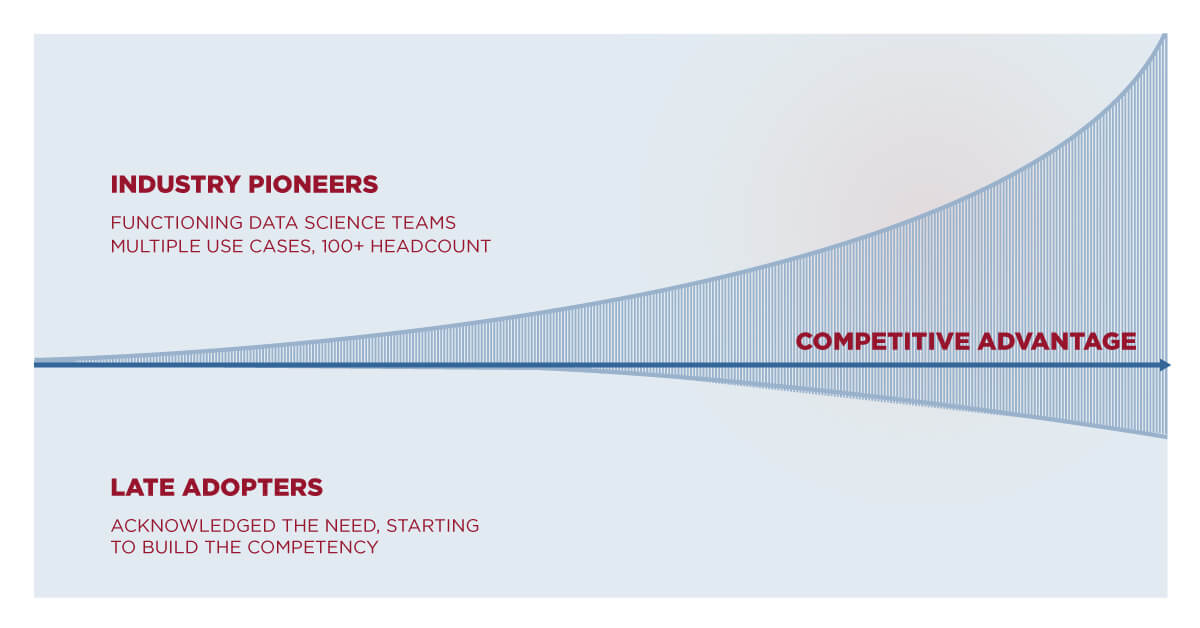
2. Workplace culture: Agile versus silos
Raiffeisenbank, TeamBank, Erste Group, Unicredit Bank—they are leading the way in agile transformation. The current trend is to break up old, inflexible silos and set up an environment suitable for the cross-pollination of ideas. Due to the nature of the banking industry, this is rarely executed for the entire institution. But the added value a data science team can bring to the table depends heavily on your cultural set-up.
At first, having a room full of able data scientists work on your problems may seem like a great idea. However, the success of a use case stands and falls with its business value and profitability. Moreover, data-based solutions permeate all departments of a bank—from marketing to risk. If your analysts don’t mix with other cohorts, their ability to come up with useful projects will be diminished.
Despite the collective vision of an ideal data scientist bridging the gap between IT and business, data scientists tend to be techies first. They are rooted in statistics and programming. In our experience, a mix of focused work within the analytics team and time spent amongst business users can do the trick.
3. Being an attractive employer
Good data scientists tend to be picky employees. Moreover, they understand very well their high demand on the job market (they love data after all). What will, however, interest them, is how good you are with your data. Do you have a data management strategy in place? Is your data structured, synchronised and harmonised? If not, then you need to get it in good shape as soon as possible. If you want to hire competent data engineers and data scientists, you need to be a data-driven organisation and vice-versa.
Finally, even with a competent team under your belt, it can be a challenge to kick-start the first few use cases and get “un-stuck” from more difficult ones. In these situations, getting external help can be the right way out. Ideally, you want a company with a strong rooting in both data science and software engineering, like Profinit.